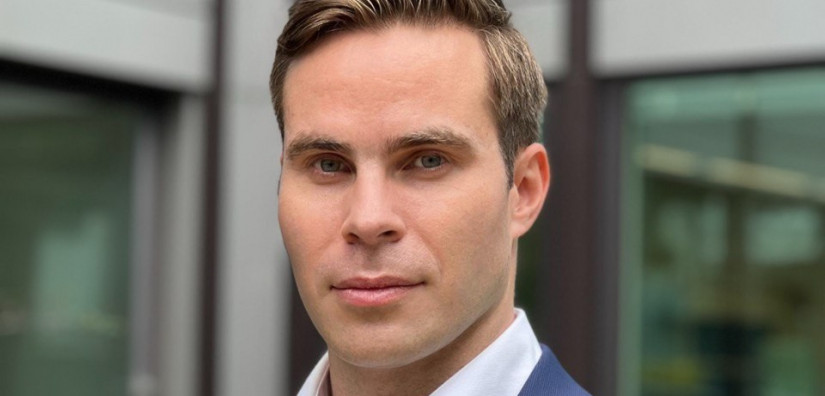
Enhancing Value-at-Risk Models with CORA and GORA: Advanced Techniques for Managing Correlation Sensitivity in Multi-Asset Portfolios
Introduction
In asset and risk management, accurately capturing correlation risk is crucial, particularly in diversified portfolios with multiple assets. Traditional risk metrics like Value-at-Risk (VaR) measure potential losses over a defined period, but they often assume static correlations. As correlation risk becomes more prominent—especially in multi-asset portfolios—advanced parameters such as CORA (correlation sensitivity) and GORA (gamma correlation sensitivity) help risk managers refine VaR models, enhancing precision and adaptability.
In this article, I’ll present CORA and GORA within the VaR framework, offering an advanced approach to risk management for portfolios sensitive to correlation. We’ll explore the mathematical foundation of these parameters and their application in quantifying and managing VaR under changing correlation dynamics.
Understanding CORA and GORA in the VaR Context
CORA (Correlation Sensitivity) and Its Relevance in VaR
CORA A measure of how much a dependent variable changes if the correlation between two or more independent variables changes by an infinitesimally small amount, in the case of a VaR model, the sensitivity of a portfolio’s loss distribution—to changes in the correlation between assets. For instance, in a portfolio with two highly correlated assets, an increase in correlation could magnify losses during adverse market conditions, directly impacting the VaR.
Mathematically, CORA in a VaR context can be defined as the derivative of the portfolio VaR with respect to correlation, ρ:
This metric is especially useful in stress testing, as it shows how sensitive the portfolio’s potential loss is to correlation fluctuations. In equation above can also be the price of a credit product such as a credit default swap (CDS) or a structured product such as a collateralized debt obligation (CDO). This is because the market price of a CDS or CDO changes when correlation changes; hence there is market price risk. This indicates the relationship between market risk and credit risk: When the market price of an asset decreases, typically the default risk increases, and vice versa.
GORA (Gamma Correlation Sensitivity)
While CORA gives the first-order effect of correlation changes on VaR, GORA measures the second-order sensitivity. It is defined as the second derivative of VaR with respect to correlation:
GORA is particularly relevant when correlations exhibit non-linear or rapid changes, helping risk managers anticipate how shifts in correlation could impact VaR in a non-linear fashion.
Mathematical Formulation of CORA in VaR
To apply CORA in a VaR model, we must consider how portfolio VaR responds to correlation changes. Let’s consider a portfolio with two assets s1 and s2 where the portfolio value V depends on the correlation between these assets. In a simple bivariate normal setup, the joint distribution of s1 and s2 can be expressed with correlation parameter ρ as:
where σ1 and σ2 are the volatilities of the respective assets.
VaR Calculation with Correlation Sensitivity
- Portfolio Loss Distribution: To calculate VaR, we model the portfolio loss distribution based on the joint distribution of s1 and s2, considering their correlation ρ.
- VaR Formula: VaR at a confidence level α is given by the quantile function of the portfolio loss distribution. For a portfolio with returns Rp and standard deviation σp , we can express VaR as:
where Φ-1a is the inverse cumulative distribution function (CDF) of the standard normal distribution, and σp is the portfolio standard deviation, which depends on the correlation ρ as:
where w1 and w2 are the portfolio weights for assets s1 and s2.
CORA for VaR
Now, differentiating VaR with respect to ρ gives us CORA for VaR:
Using the portfolio standard deviation σp formula, we compute the derivative with respect to ρ:
Substituting this into the CORA formula:
This formula shows that CORA for VaR is influenced by the weights and volatilities of the assets, as well as their correlation ρ.
Interpretation of CORA for VaR
- High CORA Value: A high positive CORA implies that an increase in correlation will result in a higher VaR, indicating that the portfolio is more sensitive to co-movements between assets. For risk managers, this signals a need for potential rebalancing to mitigate correlation-driven risks.
- Negative CORA Value: A negative CORA value would mean that increased correlation actually reduces VaR, which might occur in portfolios structured to benefit from assets moving in unison.
GORA provides a measure of how CORA itself changes with correlation, useful for assessing the stability of correlation impacts on VaR. Taking the second derivative of VaR with respect to ρ:
where the second derivative ∂2σp∂ρ2 provides a measure of convexity in the portfolio standard deviation with respect to correlation. This second-order sensitivity allows for an advanced level of risk management, particularly when correlations are highly volatile or prone to sudden shifts, such as in crisis periods.
Practical Application of CORA and GORA in VaR Models
Example: Hedging and Stress Testing with CORA and GORA
Consider a portfolio with a mix of equities and fixed-income assets. During periods of economic uncertainty, the correlation between these assets might increase. By calculating CORA and GORA for the portfolio’s VaR:
- Dynamic Hedging: If CORA for VaR is high, the risk manager may hedge against potential correlation spikes, possibly by reducing exposure to correlated assets or using derivatives that benefit from increased co-movement.
- Stress Testing: Using GORA in stress testing allows risk managers to assess how the portfolio’s VaR could change non-linearly under extreme scenarios, such as a sharp market downturn where correlations might shift dramatically.
- Portfolio Optimization: In constructing an optimal portfolio, CORA and GORA can inform asset selection, allowing managers to prioritize assets with lower correlation sensitivities to maintain a stable risk profile.
Also, please keep in mind that VaR has some limitations, especially slim tails and non-additivity, so its better to use Expected Shortfall.
Conclusion
Incorporating CORA and GORA into the VaR framework represents a sophisticated approach to correlation risk management in multi-asset portfolios. By quantifying how VaR responds to correlation changes, risk managers gain a clearer picture of how potential losses are impacted by evolving asset relationships. CORA and GORA offer flexibility for various extensions: we can compute CORA not only for an overall change in the correlation of all assets in the portfolio but also for changes in the correlation between a single asset and all other assets or changes in the correlation between two specific assets. This approach gives the risk manager a focused view of the correlation risk associated with particular assets, which could be crucial components of the portfolio, allows for proactive hedging and refined portfolio optimization strategies, enhancing resilience in dynamic markets. As correlation sensitivities become integral to managing complex risk structures, CORA and GORA serve as essential tools in the modern risk manager’s toolkit.
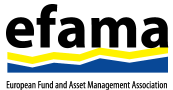
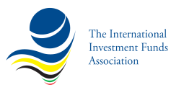
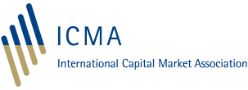